Christina Kumler
325 Broadway
Boulder, CO 80305-3328
Profile
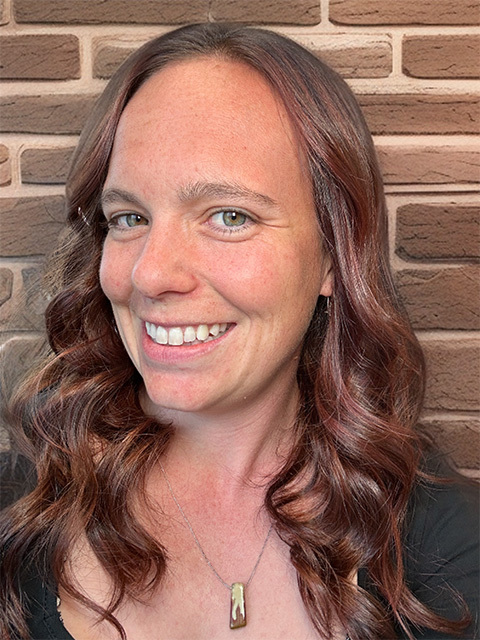
Bio:
Hello! If you're looking for someone who loves being outside and whose head is constantly looking into the clouds, then you have found her. I have many hobbies, including photography, triathlon, eating good food, and drinking good drinks. I enjoy trail running and exploring new places and cultures with my family and furry friends. Don't be fooled, though, because I also like to stay home and tinker with knitting projects or video games with friends. While I think I'm pretty balanced between all four-seasons, I'm a sucker for snow, skiing, and winter.
Research Interests:
I have most recently been working with machine learning methods, included structures like neural networks and random forests, to better utilize data we obtain from inputs like numerical weather models and satellites. I use these models to solve different problems including region of interest identification and modeling fire power into the near future. My background in applied mathematics, atmospheric, and oceanic sciences lent itself nicely to this type of work and I hope that these tools can be used to help improve forecasts, better utilize the massive amounts of data we cannot process fast enough in "real-time," and more. Additionally, I am avid about outreach opportunities. These have included a range of audiences from fellow scientists to the public; examples include teaching machine learning tutorials to college and graduate students as well as speaking about machine learning and the weather to elementary and middle-schoolers. The machine learning community in atmospheric science is growing rapidly, and I participate as the president of the Artificial Intelligence (AI) Committee president for the American Meteorological Society as well as in institutes such as AI2ES and CIRA. I lead the NOAA AI working group, which meets monthly, to discuss ongoing research, new advancements, etc. Basically, if you have an AI in atmospheric sciences question, I can either help out or point you to someone who might be able to provide more information!
Education:
- University of Miami, Miami, FL, Meteorology and Physical Oceanography, M. P. S., 2015
- University of Colorado, Boulder, CO, Applied Mathematics, B.Sc., 2013
- University of Colorado, Boulder, CO, Atmospheric and Oceanic Science, minor, 2013
Linked-In profile:
https://www.linkedin.com/in/christina-kumler-780372128/?trk=opento_sprofile_details
https://www.linkedin.com/in/christina-kumler-780372128/?trk=opento_sprofile_details
Publications:
Bostrom, A., Demuth, J. L., Wirz, C. D., Cains, M. G., Schumacher, A., Madlambayan, D., Bansal, A. S., Bearth, A., Chase, R., Crosman, K. M., Ebert-Uphoff, I., Gagne, D. J., Guikema, S., Hoffman, R., Johnson, B. B., Kumler-Bonfanti, C., Lee, J. D., Lowe, A., McGovern, A., … Williams, J. K. (2023). Trust and trustworthy artificial intelligence: A research agenda for AI in the environmental sciences. Risk Analysis, 00, 1–16. https://doi.org/10.1111/risa.14245
(Accepted and pending first round of revisions) Kumler-Bonfanti, C., R. Ahmadov, E. James, & J. Q. Stewart (2022). Short-Range Prediction of Wildfire Intensity with Random Forest Model using Weather Forecast and Satellite Fire Radiative Power Observations.
Kumler-Bonfanti, C., Stewart, J., Hall, D., & Govett, M. (2020). Tropical and extratropical cyclone detection using deep learning. Journal of Applied Meteorology and Climatology, 59(12), 1971-1985.
Bonfanti, C., L. Trailovic, J. Stewart, and M. Govett, 2018: Machine learning: Defining worldwide cyclone labels for training. 21st Int. Conf. on Information Fusion (FUSION), Cambridge, United Kingdom, IEEE, 753–760, https://doi.org/10.23919/ICIF.2018.8455276.
Lagerquist, R., J. Stewart, I. Ebert-Uphoff, and C. Kumler, 2021: “Using deep learning to nowcast the spatial coverage of convection from Himawari-8 satellite data”. Monthly Weather Review, early online release, https://doi.org/10.1175/MWR-D-21-0096.1.
Giffard-Roisin, S., Yang, M., Charpiat, G., Kumler Bonfanti, C., Kégl, B., & Monteleoni, C. (2020). Tropical cyclone track forecasting using fused deep learning from aligned reanalysis data. Frontiers in big Data, 3, 1.
Ebert-Uphoff I. Ryan Lagerquist, Kyle Hilburn, Yoonjin Lee, Katherine Haynes, Jason Stock, Christina Kumler, Jebb Q Stewart. 2021. CIRA guide to custom loss functions for neural networks in environmental sciences–version 1. arXiv:2106.09757. Retrieved from https://arxiv.org/abs/2106.09757.
(Accepted and pending first round of revisions) Kumler-Bonfanti, C., R. Ahmadov, E. James, & J. Q. Stewart (2022). Short-Range Prediction of Wildfire Intensity with Random Forest Model using Weather Forecast and Satellite Fire Radiative Power Observations.
Kumler-Bonfanti, C., Stewart, J., Hall, D., & Govett, M. (2020). Tropical and extratropical cyclone detection using deep learning. Journal of Applied Meteorology and Climatology, 59(12), 1971-1985.
Bonfanti, C., L. Trailovic, J. Stewart, and M. Govett, 2018: Machine learning: Defining worldwide cyclone labels for training. 21st Int. Conf. on Information Fusion (FUSION), Cambridge, United Kingdom, IEEE, 753–760, https://doi.org/10.23919/ICIF.2018.8455276.
Lagerquist, R., J. Stewart, I. Ebert-Uphoff, and C. Kumler, 2021: “Using deep learning to nowcast the spatial coverage of convection from Himawari-8 satellite data”. Monthly Weather Review, early online release, https://doi.org/10.1175/MWR-D-21-0096.1.
Giffard-Roisin, S., Yang, M., Charpiat, G., Kumler Bonfanti, C., Kégl, B., & Monteleoni, C. (2020). Tropical cyclone track forecasting using fused deep learning from aligned reanalysis data. Frontiers in big Data, 3, 1.
Ebert-Uphoff I. Ryan Lagerquist, Kyle Hilburn, Yoonjin Lee, Katherine Haynes, Jason Stock, Christina Kumler, Jebb Q Stewart. 2021. CIRA guide to custom loss functions for neural networks in environmental sciences–version 1. arXiv:2106.09757. Retrieved from https://arxiv.org/abs/2106.09757.