Blog Post: Untangling Positional and Structural Forecast Errors
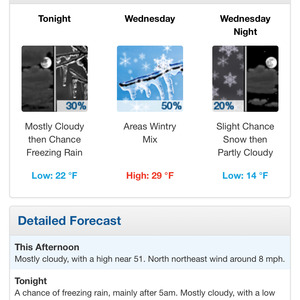
Fig. 1. Schematic for total forecast error decomposition: (1) Spatially align a forecast with the verifying analysis field; (2) Smooth original and aligned forecast and analysis to remove unpredictable smaller scales; (3) Decompose total error into orthogonal (right angle) components of (i) large scale positional error, (ii) large scale structural error, and (iii) smaller scale noise.
Numerical models use mathematical equations to reproduce the atmosphere and estimate how it evolves in time. The atmosphere, however, is chaotic and difficult to predict. Errors emerge, especially as forecasts extend out to days or weeks. Meteorologists attempt to trace these errors back to either gaps in observations, or imperfections in the models. Each type requires very different strategies to fix.
As 3-dimensional weather features like fronts and pressure systems evolve in numerical forecasts, the model has the potential to put these features in the wrong location or in a distorted shape. Researchers have studied location and structural errors (e.g., track and intensity error for hurricanes), but how they can be systematically separated for any forecast feature of interest remains an open question.
In a recent study, we used a method to divide forecast error fields into three components: large-scale positional, large-scale structural error, and smaller-scale error (Fig. 1). The method also outputs a vector field (Fig. 2) showing the displacement of the forecast compared to the observational analysis field. We found over all regions and lead times evaluated, more than 50% of the total error is associated with the weather features being in the wrong place. Distortion of forecast features such as structural inaccuracies of fronts and other weather systems is less pronounced, explaining only about 25% error variance.
Separating location and structural errors is the first step in further reducing errors in NOAA’s weather forecasts. For example, when an atmospheric river threatens torrential rain on the West coast, the landfall location can be better pinpointed by adaptively taking additional airborne observations in upstream oceanic areas. Also, errors in the geographical structure of rain can be mitigated by targeted improvements in how the model handles thermodynamic and precipitation processes.
Intrigued by the results from the present study, the authors plan to explore whether a positional-structural-noise decomposition of the spread among an ensemble of perturbed forecasts captures the same pattern as found in forecast error. A structural component in the error in excess of the spread, for example, could be quantitatively interpreted as a sign of the model missing some natural variability.
Fig. 2. 3.5-day forecast (black contour) and verifying analysis (shades of color) of mean sea level pressure for Hurricane Katia, valid at 12 UTC 6 September 2011. Moving the forecast along with the blue arrows aligns it with the observational analysis.
Dr. Isidora Jankov is a branch chief at the Global Systems Laboratory (GSL) with NOAA Research. Her research is focused on ensemble-based data assimilation, model physics, the design of ensemble forecasting systems, and ensemble statistical post-processing, with an emerging emphasis on Machine Learning algorithms.
Dr. Zoltan Toth is a Senior Scientist at the Global Systems Laboratory (GSL). His research has covered topics in atmospheric and related dynamical systems, including the dimensionality and equilibrium properties of the climatic phase space, the limits of predictability, ensemble forecasting, theoretical and practical aspects of data assimilation, uncertainties in numerical modeling, sensitivity studies, and the statistical calibration and socio-economic use of weather forecasts.
Citation: Jankov, I., S. Gregory, S. Ravela, Z. Toth, and M. Peña, 2021: Partition of Forecast Error into Positional and Structural Components. Adv. Atmos. Sci., https://doi.org/10.1007/s00376-021-0251-7.
Hoffman, R. N., Z. Liu, J. Louis, C. Grassotti, 1995: Distortion representation of forecast errors, Mon. Weather Rev., 123, 2758-2770.
Keil, C., & Craig, G. C. (2009). A Displacement and Amplitude Score Employing an Optical Flow Technique, Weather and Forecasting, 24(5), 1297-1308. Retrieved Jan 11, 2022, from https://journals.ametsoc.org/view/journals/wefo/24...
Ralph, F. Martin, Michael D. Dettinger, Jonathan J. Rutz, and Duane E. Waliser (Editors), 2020: Atmospheric Rivers, pp. 252. DOI https://doi.org/10.1007/978-3-030-28906-5
Sivillo, J. K., Ahlquist, J. E., & Toth, Z. (1997). An Ensemble Forecasting Primer, Weather and Forecasting, 12(4), 809-818. Retrieved Jan 11, 2022, from https://journals.ametsoc.org/view/journals/wefo/12...