2023 Summer Intern Projects
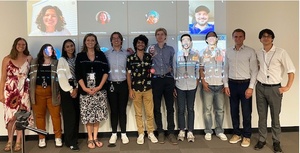
GSL hosted 14 interns this summer! Four graduate and seven undergraduate students were funded by a GSL and the Cooperative Institute for Research in Environmental Sciences (CIRES) Professional Research Experience Program (PREP-GSL/CIRES). This is the second year of PREP-GC and the first year to host interns in person! Three undergraduate students were funded through the NOAA Office of Education. All interns presented their summer research on August 2. The titles and summaries are below!
Reagan (Professional Research Experience-GSL/CIRES (PREP-GC): Reagan is a rising Sophomore at the University of Oklahoma pursuing a degree in Meteorology. Reagan hopes this time in the GSL Internship Program will bring lots of learning and networking that will benefit her as she continues her undergraduate degree. She is excited to meet some amazing people who are interested in the same things.
Mentors: Dave Turner, Laura Bianco, Irina Djalalova
Project: Evaluating the Ability of the HRRRv3 and HRRRv4 to Forecast Wind Ramp Events in the Great Plains
Integrating energy produced by wind turbines into the electrical grid efficiently requires better knowledge of how the wind resource may change with time so that utilities can be prepared to meet the shortfall (if the anticipated wind decreases dramatically) or scale back thermal generation (if there is more wind than anticipated). Thus, accurate forecasts of intense changes in wind speed over short periods of time are critical. This project will evaluate the ability of the operational High-Resolution Rapid Refresh version 4 (HRRRv4) compared to the older version (HRRRv3) to capture wind ramp events across the Great Plains, and how the accuracy might depend on forecast timing, location, or other factors.
Paulina (PREP-GC): Paulina is a rising senior from Santa Clara University in Environmental Studies. She wanted to try something new this summer, learn new skills, and meet new people. She has always been interested in learning about the work done at NOAA, and is very excited for the opportunity to explore a new city in her home state! She will be modifying configuration files in a simulation of idealized slope flows to run new numerical experiments.
Mentors: Duane Rosenberg, Isidora Jankov
Project: Numerical Study of Katabatic Flows with a Density Current Source
Local weather such as visibility, fog, and air pollutants can be influenced by cool, dense air moving downhill towards warmer, less dense air known as katabatic winds. Katabatic flows also occur on a larger scale, for instance, in Antarctica and Greenland, and contribute to the state of weather and climate of these global regions. Paulina will be working on configuring, running, and analyzing numerical simulations of idealized katabatic slope flows driven by a density current.
The numerical experiments will reflect changes in the boundary conditions, in regards to the angle of the slope and the thermal flux of the slope surface. This will allow for the computation of new solutions to the equations that will yield a variety of outcomes to help explain the evolution of these interesting flows in nature. Visual analysis, in the form of movies to better understand temporal behavior, and figures restricted to one or more snapshots to examine spatial behavior, will aid in developing a more detailed understanding of these flows in even less idealized scenarios.
Jagger (PREP-GC): Jagger received his BA in Mathematics at Vanderbilt University and MS in Climate and Health at the University of Miami. He is currently pursuing a PhD in Geosciences at the University of Texas at Austin with a focus on applying models to public health prediction. Jagger joins WIDS working to add human risk information into DESI.
Mentors: Travis Wilson, Rob Howlett
Project: Hazard Vulnerability and Impact Visualization
It is crucial that stakeholders, including forecasters who issue alerts, watches, and warnings, policy-makers, and other decision-makers, understand how forecasted weather could impact human populations. DESI (Dynamic Ensemble-based Scenarios for IDSS), developed by NOAA GSL, is a decision support tool that provides forecast information based on multiple models. Displaying human risk information, such as the CDC Social Vulnerability Index, will add significant value to DESI by allowing decision-makers to simultaneously view a forecast and the geographic extents of various populations. In addition, displaying historical hazard information from NOAA NCEI Storm Data and other sources would give decision-makers the ability to view historical weather hazards, their magnitudes, and associated damages for any given area. During the PREP-GC program, I hope to strengthen DESI by adding in these features among others, with the ultimate goal of helping decision-makers to best utilize weather forecasts to protect human populations.
Lindsey (PREP-GC): Lindsey is currently an Analytical, Environmental, and Atmospheric Chemistry Ph.D. candidate at CU Boulder. Lindsey joins EPAD to study how using different emission ratios for wildfires in different combustion stages (flaming vs. smoldering) impact HRRR-Chem model predictions.
Mentors: Ravan Ahmadov, Jordan Schnell
Project: Using Hourly Wildfire Potential and Satellite Data to Estimate the Impact of Changing Combustion Conditions on the Composition of Wildfire Emissions
he buildup of fuel from fire suppression practices has increased the number, size, and intensity of wildfires. Current air quality forecasts use vegetation type and area burned to estimate the composition of wildfire smoke. However, laboratory and field studies have shown that the chemical composition of wildfire smoke also depends on whether the fire is flaming or smoldering. Previously, we showed that remote sensing can be used to study how the chemical composition of wildfire smoke changes over time, as wildfires progress from more flaming to smoldering. Allowing the chemical composition of wildfire smoke to change with evolving combustion conditions could improve air quality forecasts. During the PREP-GC program, we found a relationship between Hourly Wildfire Potential, derived from HRRR-Smoke, and remote sensing observations that we will use to inform how the chemical composition of wildfire smoke should change over time.
Eli (PREP-GC): Eli joins us from St. Cloud State, working in EPAD. Eli applied to the CIRES / GSL Internship Program because he is interested in a research career and is curious about the work done at GSL. Eli loves the mountains and is excited to meet a bunch of like-minded scientists and students this summer.
Mentors: Ben Green, Shan Sun
Project: Seasonal Unified Forecast System Performance with a focus on El Niño
Seasonal forecasting is important for predicting changes in large-scale weather patterns months in advance. One application of this is forecasting El Niño, which has significant implications for weather patterns across the globe. One of the strongest El Niño’s on record occurred during late 2015 into 2016, likely contributing to a severe drought in the Caribbean and unusually active hurricane season in the Pacific. This project explores how well the Unified Forecast System (UFS) does in predicting El Niño, which is determined by looking at sea surface temperature anomalies in the central and eastern Pacific. Results indicate that the UFS captured the strong El Niño of 2015 but has significant challenges predicting sea surface temperature, cloud cover, precipitation, and other variables at a seasonal time scale.
Sarah (PREP-GC): Sarah is a recent graduate from Metropolitan State University of Denver with a B.S. degree in Meteorology, and a minor in
Mathematics. Sarah participated in a Research Experience for Undergrads (REU) at CU Boulder last summer, which inspired her to dive deeper into Python coding, scientific research, and thinking about graduate school. As Sarah began to learn more about doing research and using python for data analysis, she realized how much she enjoyed the research Process. Sarah is a proud mother of two children and two fur-children. Her talents include playing the cello since the age of 7, and she likes to sing and play music in her free time.
Mentors: Gerard Ketefian, Jeff Beck, and Ligia Bernardet
Project: Using the Short Range Weather App and the Grell-Freitas Convection Scheme to Analyze the Mesoscale Convective Complex of June 24-25, 2023
One of the great challenges of forecasting the weather in high resolution is trying to account for the effects of small but thunderstorm clouds within a grid cell. A numerical weather prediction technique known as convective parameterization helps address this challenge. This research evaluates the impact of a particular convective parameterization scheme on short-range weather forecasts using two different physics suites. One suite uses the scheme, the other does not. This research will analyze how the scheme affects precipitation and reflectivity threshold forecasts. We also ran a verification tool on the model output and plotted the tendencies of temperature averaged over a specific forecast hour to see how the parameterized variables are affecting the forecast.
Robby (Lapenta): Robby was selected as an intern at GSL through the NOAA Lapenta Program. He joins us at the completion of his Junior year at the University of Oklahoma School of Meteorology. Robby has spent time working with numerical modeling outside of class and feels he'll fit right in! He is excited to spend time in Colorado this summer and be in a new work environment. Robby plans to go to graduate school and feels this experience will prepare him for the change that comes with becoming a graduate student.
Mentors: Jeff Beck, and Ligia Bernardet
Project: The Impacts of the Grell-Freitas Scheme on Short-Range Forecasts of the April 19, 2023 Convective Event
The Unified Forecast System (UFS) is a community-based earth modeling system that includes the Short Range Weather (SRW) application and serves as the foundation for NOAA’s next-generation Rapid Refresh Forecast System (RRFS). Robby is working on getting the UFS and SRW application ready for use in the RRFS. A known problem with the RRFS is that it predicts too many thunderstorms. His project was to run the SRW app on the April 19, 2023 severe weather outbreak in central Oklahoma, which was difficult for current weather models to predict. I ran the app with and without modifications to model calculations that may help improve how the model predicts clouds and precipitation. My results show the modified forecast model fixed some of the problems with over-prediction of thunderstorms, creating a more accurate forecast of severe weather in Oklahoma. Results like this may aid model developers with improving the RRFS, adding new tools to the arsenal of operational forecasters in the future.
Sean (PREP-GC): Sean is currently a graduate student at CU Boulder studying applied math with a concentration in data science and statistics. Mentors: Jana Nasciemento, Haiqin Li and Isidora Jankov
Project: Applying the Visible Energy Fraction to Investigate Nighttime Variations in Fire Combustion Phase
There has been an increased incidence and severity of wildfires in the last several decades. The effects of wildfires are far-reaching, as the burning of vegetation and biomass causes the emission of various greenhouse gasses and particulate matter into the atmosphere, which scatter and absorb solar radiation and air quality far beyond the localized burn area. Accurately estimating emissions from wildfires is challenging in part because the amount of generated byproducts depends on the fire combustion phase (i.e. if the fire is actively flaming or smoldering). I will be working on better characterizing the combustion phase of wildfires in our existing emissions forecasting models and comparing these new emissions estimates in historical wildfire cases.
Kevin (PREP-GC): Kevin is a second-year Intern from Florida IT with a major in Aviation Meteorology. He will continue his work in WIDS on the Weather
Archive and Visualization Environment (WAVE).
Mentors: Jebb Stewart, Paula McCaslin
Project: Weather Archive and Visualization Environment (WAVE) Improvements
WAVE is a multi-purpose web-based system that effectively manages and visualizes meteorological data. WAVE allows NOAA National Weather Service (NWS) forecasters to create consistent, understandable graphics for use in decision support, on social media, and in presentations. Decision support and guidance for the public is a critical service provided by the NWS. This project aims to improve current datasets, incorporate point-based observational data, and fulfill user feature requests.
Blake (PREP-GC): Blake joins us from the University of North Dakota and will be working in WIDS. Growing up on a farm in North Dakota sparked his interest in weather, as he could see from a young age the impacts severe weather can have on people. Before his time here, Blake interned at a TV station in Fargo and volunteered at the NWS FGF office. Both of these opportunities further built his interest in forecasting, but also in the social science aspect of meteorology. His goal in a future career is not only to be a great forecaster but also to be able to find and develop the best methods of communication so that personal decisions the public makes are based on information they can easily understand.
Mentor: Cole Vaughn
Project: Improving Graphical Communication of Winter Weather
Winter storms routinely interrupt daily life across much of the Northern United States. Recently, the Winter Severity Index, along with the use of probability forecasts, has become mainstream in public messaging. However, there is concern that probabilistic forecasting is too complicated for the public. The goal of this project is to determine how well the public understands winter probabilistic and impact-based forecasts, as a great forecast with poor messaging will still lead to incorrect decision-making. To determine how winter impacts are interpreted by the general public and the forecasters who produce them, surveys will be conducted to find areas where we can improve our messaging. Results will also be compared against traditional snowfall forecasts to glean input from the public on which products are easier to understand and most importantly, help them make the most informed decisions when preparing for winter weather impacts.
Andres (PREP-GC): Andres is from San Juan, Puerto Rico, and attends the University of Puerto Rico in Rio Piedras. He has a B.S. in computer science with a minor in mathematics. He is now pursuing a graduate degree in Earth and Environmental Sciences.
Mentors: Sean Murdzek, Terra Ladwig
Project: RRFS vs HRRR: An ML-based model verification approach for severe winds
The Rapid Refresh Forecasting System (RRFS) is a prototype single model, convection-allowing, weather forecasting system that is set to become operational as of early 2024. This model is part of NOAA’s effort to unify and simplify the modeling suite based on the FV3 model. In this research project, we use the RRFS model outputs from the 2023 Hazardous Weather Testbed Spring Forecasting Experiment to evaluate the model performance in predicting severe convective winds. We use an approach similar to Sobash et al. (2019) where NOAA Severe Wind Reports are used as ground truth and are used to evaluate model performance. We are also developing software tools to simplify data management and to perform these evaluations.
Ev (Lapenta): Ev is a rising senior at Valparaiso University who was selected to participate in the William M. Lapenta internship program at GSL!
Mentors: Jeff Duda,Terra Ladwig
Project: A Comparison of MRMS and GREMLIN Composite Reflectivity using the Method for Object-Based Diagnostic Evaluation
The Multi-Radar Multi-Sensor system (MRMS) is a product that incorporates information from weather models, observed weather conditions, and radars in the United States and Canada. In addition to its role in forecasting, it has also found other uses, including its incorporation into weather models, where the accuracy of the initial data is critical for the accuracy of the model’s forecast. However, MRMS has gaps in coverage in mountainous terrain, over open water such as the Gulf of America (formally Gulf of Mexico), and in regions that lack reliable radar coverage. One proposed solution is the use of satellite-derived products, which lack these limitations. One such product is GOES Radar Estimation via Machine Learning to Inform NWP (GREMLIN), which estimates radar reflectivity from satellite observations. This project uses an object-based comparison tool to evaluate GREMLIN’s performance compared to MRMS, and therefore, its potential to supplement or replace MRMS where MRMS data is typically used.
Jeff (PREP-GC): Jeff joins us from OU and will be working in WIDS in the area of model validation. Jeff first became interested in STEM through programming in robotics. He is interested in machine learning and data science and wants to apply his skills in atmospheric science.
Mentor: Hongli Wang
Project: PurpleAir PM2.5 Quality Control and Bias Correction for Data Assimilation
The Rapid Refresh Forecast System, or RRFS, is a next-gen “convection-allowing, ensemble-based data assimilation, and forecasting system” model that will also include a smoke product. The RRFS smoke model will be an evolution of the HRRR smoke model and continue to offer high-resolution, short-term forecasts of smoke. The RRFS smoke model offers several improvements over that of HRRR, one of which is the inclusion of air quality sensor data for both initial conditions and data assimilation. Two networks of air quality sensors in the U.S. are widely in use now: AirNow and PurpleAir. AirNow sensors follow stricter quality control standards compared to PurpleAir sensors. However, PurpleAir sensors are much more numerous, as they are cheaper and easier to install. The goal of my work is to develop a method to correct the PurpleAir data by comparing them to collocated AirNow data. This will allow for more consistent input into the RRFS smoke model.
David (Hollings) Princeton University
Mentor: Stephen Weygandt, Amanda Back
Project: The robustness of lightning proxies for numerical weather prediction and data assimilation
The Geostationary Operational Environmental Satellite-R (GOES-R) East and West satellites launched in 2016 and 2017 provide a high detection efficiency lightning detection system. Flash extent density, the number of flashes occurring within a period of time at a given location, can be calculated to produce a high spatial and temporal resolution database. While radar is a vital tool used in data assimilation in numerical weather prediction models, limitations result in lacking data across mountains, oceans, and dead zones. The system can detect lightning without such coverage limitations and has led to the exploration of its viability in data assimilation. This study explores the applicability of candidate parameterizations of flash extent density to events including thundersnow, tropical cyclones, and mesoscale convective systems. This study aims to provide important insight for improvements in flash extent density, parameterizations and assimilation.